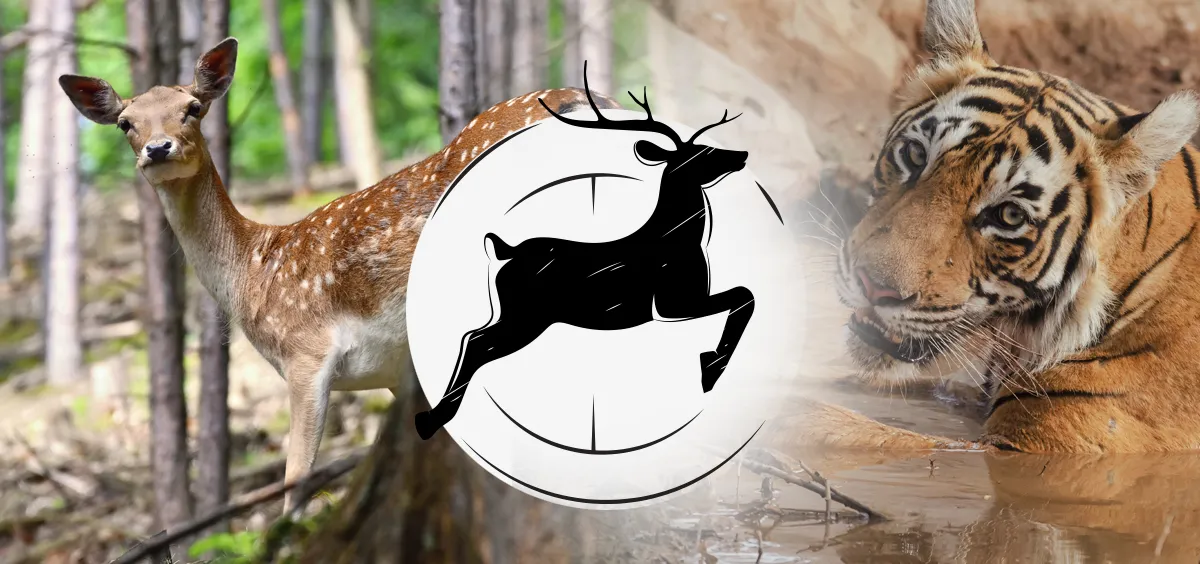
Poaching and illegal smuggling of wildlife have remained a cause of concern for wildlife authorities. As per the World Wide Fund for Nature (WWF), Wildlife trade poses the second-biggest direct threat to the survival of species after habitat destruction. As per the study conducted by TRAFFIC, a leading wildlife trade monitoring networking of the World Wide Fund for Nature (WWF), around 1,11,312 individual tortoises or freshwater turtles (11,000 a year) have been illegally traded across India since 2009. While several organizations and regulatory authorities are trying to curb the incidences of poaching, the poachers seemed to have always remained one step ahead of the patrollers.
Prof. Milind Tambe’s research group at Harvard University had been working on this problem for quite a while. Prof. Balaraman Ravindran, who is a mind tree faculty fellow and professor at IIT Madras and also heads Robert Bosch Centre for Data Science and Artificial Intelligence (RBCDSAI), looked at Prof. Tambe’s research in the area and found a scope of improvement to make it more efficient. He along with his research group decided to get together with Prof. Tambe’s group to find a better solution to this problem. The research team realized that resource allocation of the rangers and drones together with the coordinated patrolling with communication in real-time can be a good strategy to protect conserved areas with wildlife, however, none of the earlier models developed for the task included both these components. Therefore, they decided to develop such a model and algorithm that considers both of the above points.
“The work is motivated by the need to perform strategic resource allocation and patrolling in green security domains to prevent illegal activities such as wildlife poaching, illegal logging and illegal fishing. The resources we consider are human patrollers (forest rangers) and surveillance drones, which have object detectors mounted on them for animals and poachers and can perform strategic signalling and communicate with each other as well as the human patrollers,” explains Prof. Ravindran.
The efforts of the research team paid off as they have finally come up with a novel algorithm named “CombSGPO” (Combined Security Game Policy Optimization) which provides defenders with a resource allocation strategy of drones and human rangers along with a patrolling strategy for protecting wildlife.
In the past, researchers have developed models based on game theory to protect wildlife. Game theory tries to predict how a player in the game will choose among different strategies when the outcome of the situation depends on how everyone else in the group behaves. In context of wildlife protection, the game theory pertains to predicting the areas where poaching may take place. These predictions are based on the earlier poaching incidents and the interaction between poachers and defenders.
“The game model and the kind of resources we use to simulate such a “poaching game” between the defender (patrollers + drones) and attacker (poachers) are based on the widely studied Stackelberg Security Game model and are linked to drones that have already been deployed by Air Shepherd,” adds Aravind Venugopal who is a Post baccalaureate Fellow at RBCDSAI and first author of the study.
Prof. Ravindran’s team developed a model based on game theory that works in two steps. Firstly, it handles resource allocation and in the second stage it strategizes patrolling after the allocation has been done. This game model developed by the team is being used for CombSGPO algorithm. This algorithm is based on prior information of the animal population in the conserved area and also assumes that poachers have an idea about the patrolling being done at various sites. The team compared this new algorithm with the similar existing tools and found that their algorithm provides with better strategies and is more scalable than the earlier ones.
Excited with this feat, the team looks forward to making strategic patrolling capable in AirSim (a 3D environment), to use images instead of binary input and also to perform sample-efficient multi-agent reinforcement learning to learn with the least amount of data since data collection is costly in a real-world scenario. They believe, if this is achieved, we will be able to deploy multi-robot system in the real world, and perhaps in a variety of different domains like security, search and rescue, aerial mapping for agriculture etc.
Contributors
Aravind Venugopal, Elizabeth Bondi, Harshavardhan Kamarthi, Keval Dholakia, Balaraman Ravindran, Milind Tambe
Article
Venugopal, A., Bondi, E., Kamarthi, H., Dholakia, K., Ravindran, B., & Tambe, M. (2021). Reinforcement Learning for Unified Allocation and Patrolling in Signaling Games with Uncertainty. In Proceedings of the 20th International Conference on Autonomous Agents and MultiAgent Systems (pp. 1353–1361).(AAMAS 2021)
Keywords
Multi-agent Reinforcement Learning, Wildlife protection, Poaching, Patrolling