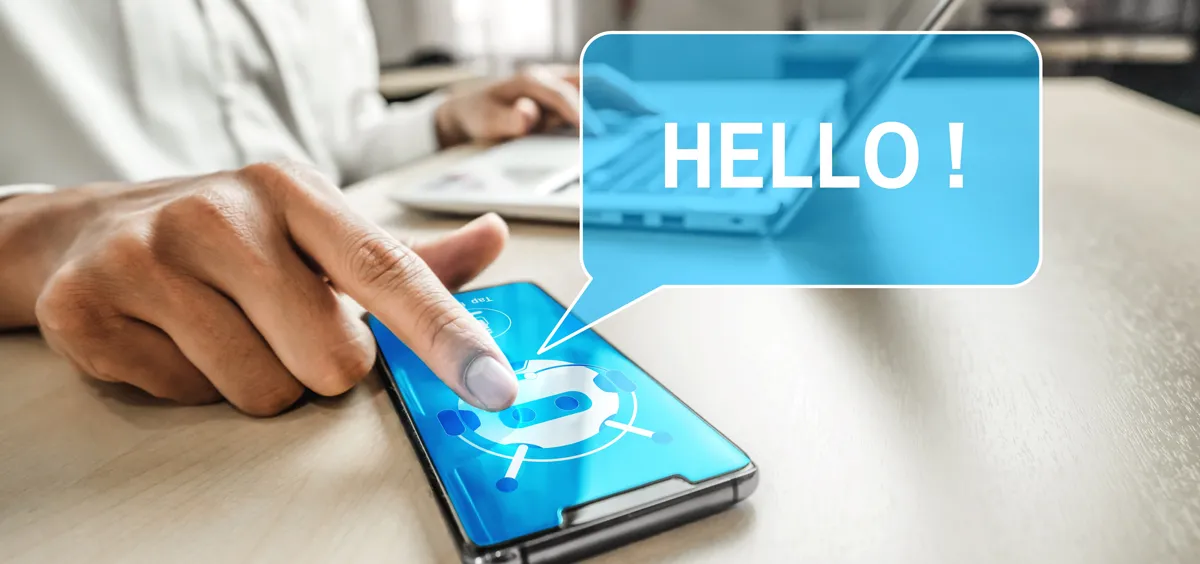
How are you feeling today? Good, Great, fine, bad, worst, bored? Either way, you are feeling, you will agree that there is no one right answer to that question and many other millions of questions where multiple answers are correct. While you, as a human, would understand that for some questions there is no single right answer, it’s difficult to teach this to machines! This is one of the problems AI researchers are struggling with today, especially in the chatbot and virtual assistant space. This is because the AI models designed for dialogue applications, like any other AI model, are trained on a dataset that has a single answer to a single question.
Dr Mitesh Khapra’s group at RBCDSAI, IIT Madras have worked around this problem and proposed an enhanced version of a dialogue dataset called DailyDialog++ as the solution. DailyDialog++ is an extension of the DailyDialog dataset which has five additional relevant and irrelevant responses each for 11000 contexts in DailyDialog. Researchers believe that this dataset will help in better training of dialogue models.
“While several datasets are used for training dialogue generation models, in this work, we show how the DailyDialog++ dataset can be used to enhance / train automatic evaluation metrics for dialogue evaluation. This can be further augmented by pretraining the metric using large-scale data such as conversations on Reddit. We also show that all the existing metrics are unable to reliably classify or score the adversarial responses in the dataset, and the potential for future work on these lines,” says Ananya B. Sai, a research scholar at RBCDSAI, IIT Madras and one of the first author of the study.
The quality of the conversation by various dialogue systems, chatbots, and virtual assistants like Siri, Cortona, Alexa etc., is measured by evaluation metrics. However, the evaluation metrics themselves need to be robust and reliable to correctly understand the scientific progress made in such applications / research directions. The researchers decided to check the performance of various evaluation metrics using this newly developed dataset. They found that n-gram based metrics and embedding based metrics were not very good at separating relevant responses from random negatives and although model-based metrics were good in this aspect, they were not good at evaluating adversarial statements. Therefore, the team developed a new BERT based evaluation metric called DEB which is pretrained on 727 Reddit conversations. Though DEB was found better than other existing models on separating random negatives but wasn’t good in identifying adversarial responses.
“The rapid pace at which dialogue models are trained and proposed makes human evaluations both expensive and time-consuming. Hence automatic evaluation metrics are widely used by the NLP community. This work is a step towards developing more reliable automatic evaluation metrics, by adopting strategies like large-scale pretraining and by improving the existing resources with adversarial examples for challenging the metrics, and multiple references for helping propose better metrics,” adds Ananya while specifying the real-world applications of the study.
Contributors
Ananya B. Sai, Akash Kumar Mohankumar, Siddhartha Arora, Mitesh M. Khapra
Article
Ananya B. Sai, Akash Kumar Mohankumar, Siddhartha Arora, Mitesh M. Khapra, Improving Dialog Evaluation with a Multi-reference Adversarial Dataset and Large Scale Pretraining. In Transactions of the Association for Computational Linguistics 2020; 8 810–827.
Keywords
Dialogue models, Chatbots, BERT, Adversarial statements, Automatic evaluation metrics