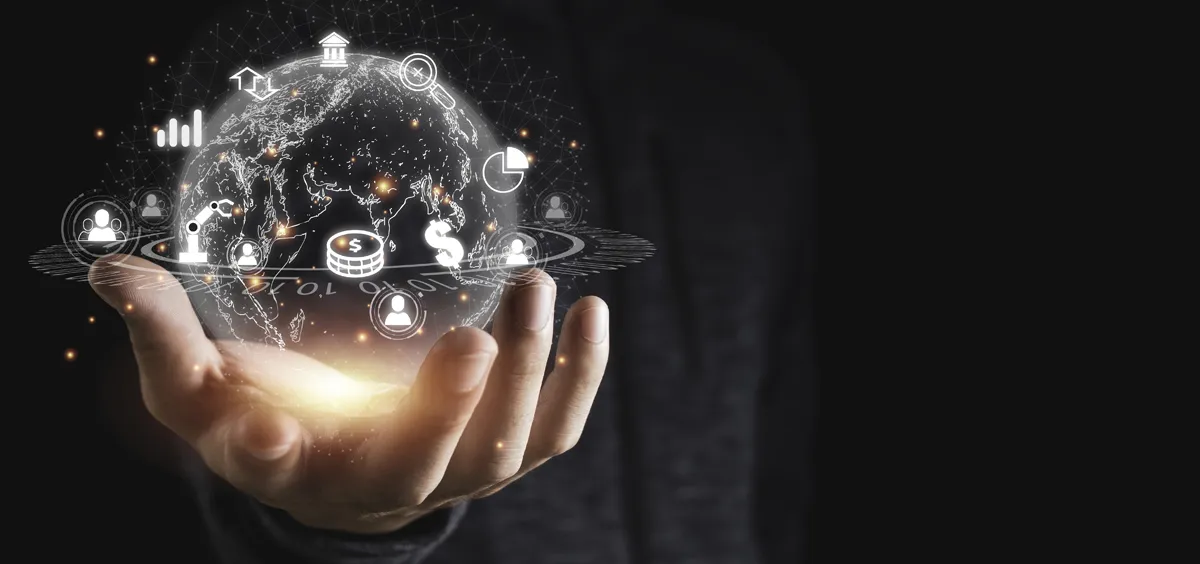
Undoubtedly, loans are one of the major sources of income for banks and other related financial institutions. A loan helps the customers to meet their short term financial needs and the interest given by the customer is the way a bank makes a profit. At once it may seem that extending more loans can increase the profit of such financial institutions, however, laxity in granting loans can lead to more losses as many customers may default on the loan repayment. Therefore, a robust prediction mechanism that can foretell whether a customer will be able to repay his/her debt is vital for financial institutions to avoid losses. These defaults are much more difficult to predict in microfinance sectors that cater to rural populations or individuals who do not have access to banking facilities as these customers do not have a credit history.
Till now, determining delinquency in loan payment has remained the goal of prediction models. However, many financial institutions now feel that the financial distress of a household is a more robust indicator and should be predicted or measured while granting a loan. This is because the financial well being of a household is already in poor shape before it ultimately defaults a loan repayment. Therefore, if the financial well being of a household is predicted one can predict that they are going to default loan repayment with more confidence. However, predicting financial distress or the over-indebtedness of a household is a much more daunting task than predicting delinquency as it depends on a myriad of factors. Dr Nandan Sudarsanam and his team, consisting of Kaushik Bhat and Anusha Kumar, at IIT Madras have now been able to develop a framework that can be used to detect over-indebtedness of a household.
For this study, the team utilized the financial data of 200,000 households over three years collected by a leading financial service provider in the under-banked sector in India - Dvara KGFS. The data contained details about assets, liabilities, income, expense, and household’s financial goals of these households. The households which did not pay their EMIs for more than 5 days then their due date were tagged as delinquent for that particular quarter in the study.
The team hypothesized that delinquency of loan repayment can be considered as a subset of overindebtedness and therefore it is important to first establish the relationship between delinquency and overindebtedness.
To establish this relationship, they used a sequential modelling approach based on Positive and Unlabeled (PU) learning. Using this approach, the households that defaulted on loan repayment or EMI were considered positive class as they are financially stressed whereas other households are considered in unlabelled class as it was not definite that they are financially sound or stressed. Owing to the temporal nature of the data, Recurrent Neural Networks (RNN) is used to predict the future delinquency status of a household. The team used a new cost function in the RNN model to improve the predictive accuracy while predicting financial distress.
The team validated their prediction framework on a Dvara survey conducted on 400 households for one-year data. The USP of the framework is that it can determine the financial stress of a household even when they are making their EMIs. Now that this framework has been developed, the microfinance sector can use it to understand the financial well being of their customers to lend loans more smartly.
Contributors
Dr Nandan Sudarsanam, Kaushik Bhat and Anusha Kumar
Keywords
Loan, positive and unlabeled learning, neural network, Artificial intelligence, microfinance, biased RNN