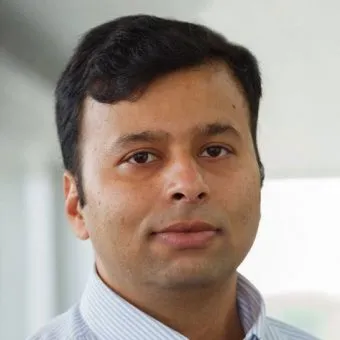
-- Dr. Sunandan Chakraborty --
The web is a sea of information where the much-needed information may just seem a click away! However, mining exhaustive information from the web is not a trivial and straightforward task as it looks to be. Therefore, AI researchers are developing natural language processing models to mine relevant information from the web. One such researcher is Dr. Sunandan Chakraborty, Assistant Professor at the School of Informatics and Computing at Indiana University. Sharing his research work, he gave a talk entitled “Extracting cues of causality from Medical text to mine factors related to Sjögren’s Syndrome” on 28th July at IIT Madras. The talk was hosted by RBCDSAI.
Dr Sunadan started by talking about the vision of his research which entailed building machine learning and Natural Language Processing models to search for information from a variety of online data such as online news, social media blogs, scholarly articles, e-commerce, online education materials and reports etc. He further said that he is particularly developing such models to address problems which either have social (Health, education, microfinance) or economic impact (food prize, interest rate, illegal wild trade). Next, he talked about an auto-immune condition named Sjögren’s syndrome where tear and saliva-producing glands are destroyed by the body’s immune system causing symptoms like dry eyes, dry mouth and joint pain. This is the reason that patients visit different specialists- ophthalmologists, dentists, and rheumatologists to treat the symptoms and a specialist isn’t able to identify the problem hence diagnosis takes a very long time. Major issues in the diagnosis of Sjögren’s syndrome include no comprehensive knowledge base on factors, symptoms, risks; the fact that the common symptoms of the disease are shared by many diseases, spread of patient data across different sources and the lack of communication between dentists and physicians.
Dr Sunandan said that the solution to such a problem is to extract the information from the scholarly article about this disease, however, this information about the disease is often expressed as causal sentences. Therefore, he decided to develop models that can extract and infer causality from the text. He told that such an idea emerged from the volatility in the onion price graph. He next explained that the onion price is affected by heavy rainfall, truck strikes, transportation costs, export and import decisions of the government and many more. They wanted to efficiently mine events from news articles and use them as features to predict onion prices so they figured out that events from news articles are represented by a certain number of verbs called event triggers. He further said that a news article has both main event trigger and subsidiary triggers apart from location and time associated with the event. They also found that each event class such as protest, election, crime, accident etc is represented by event trigger words such as demonstrate, agitate, strike represents event class protest. They decided to build such classes in their study and made various assumptions to do the same. Dr Sunandan’s team developed a model from a ten year news corpus which had seven lakh news articles and six lakh unique words. In this event-driven predictive model, the prediction was made using event-driven recurrent event newtork (REN) and inputs were drawn from the event time series. The analysis was done on the prices of eleven food commodities and it was found that their event ARIMA model is better than other models. Similarly, they also used their model for predicting the cryptocurrency values and for predicting stock prices and found that though their model could predict the trend but not actual values.
Next, they sought out to find out out of reason of various events for example which event is responsible for onion price rise but found that such an analaysis is not scalable. They found that if they draw time series of words and do a correlation they get interesting trends for example the frequency of word malaria goes up in news media 2 months after the frequency of word monsoon or floods goes up in the news media . Such trends begs question that if flood or monsoon is causing malaria. They tested this using Granger Causality tests where the input is the normalized time series of term frequency counts of words, bigrams in news text and output is the sparse graph with link between terms. If a constant unidirectional time lag is seen between the surge of two words then one can be considered cause of other. Next, they created a causal network of unigrams and bigrams from the news media. They also used the predictive causal links in news to predict stock prices and the results were encouraging as they were further able to predict factors from news that influenced the stock price.
Next, they aimed at extracting the causal relationships from the text data to predict event that might lead to a change in the future. Therefore, they decided to work on extracting entities corresponding to cause and effect respectively from a single sentence. For this, they had to identify phrases belonging to each class and then sought to find the cause entity, effect entity and the connector between them, however, there were various challenges. for example one sentence can be expressed in various ways i.e. sentences with causal meaning may not be explicit. Some other major challenges in achieving this target included multiple cause-effect pairs, irregular pattern in sentences.
To solve this problem they used datasets SemEval and Adverse drug effect (ADE) and also developed a PubMed abstracts database containing 18000 sentences which wereabout Sjögren’s syndrome. Their methodology included a reinforcement learning model and results showed that their approach was better in terms of precision, recall and F1 score than LSTM- Glove, BILSITM- Glove and BERT models in both SemEval and ADE datasets in direct evaluation. In Indirect evaluation i.e. Question answering, their approach had a precision rate of 0.864 in identifying the correct cause phrase from the question and when the identified causal phrase was correct then in 95% of cases the model was able to identify the effect correctly.
Next, they applied this new model in diagnostic issues related to Sjögren’s Syndrome. The bigger picture here was to merge and match the factors associated with Sjögren’s Syndrome in medical research articles with entries extracted from the electronic health record and electronic dental record to derive a longitudinal Sjögren’s Syndrome patient profile. Here the major challenges were on the annotation front as it required skilled annotators. So, they collected a dataset from scholarly articles and found around 25000 sentences related to Sjögren’s Syndrome, annotated 1058 sentences and used 383 causal sentences for testing. Their causal factor extractor worked by classifying a sentence as causal or non-causal and then carrying out causal relationship extraction using model pre-trained on SemEval and ADE datasets to derive signs, symptoms and associated conditions. The team compared their approach to other models which were trained to do entity recognition for any biomedical text such as Bi-LSTM, Glove Embeddings+CNN, BioWordVec+CNN, BioBERT and Gram-CNN and found that their method outperformed all of them.
Highlighting the major limitations of the study, Dr Sunandan said that though their approach can identify more than two factors it cannot label more than two factors, the approach also assumes that Sjögren’s Syndrome will appear in the sentence which may not be the case. Lastly, sometimes signs, symptoms and associated factors may appear in non-causal context which the approach cannot identify. Elaborating on the future directions of his work he said that his lab wishes to develop models to explore other relationships to extract other labels and also models that can quantify the strength of a relationship. The talk piqued the interest of the audience and an exhaustive discussion followed after the talk.
The video is available on our YouTube channel: Link.
Keywords
Natural language processing, Reinforcement learning, Text mining, Causality