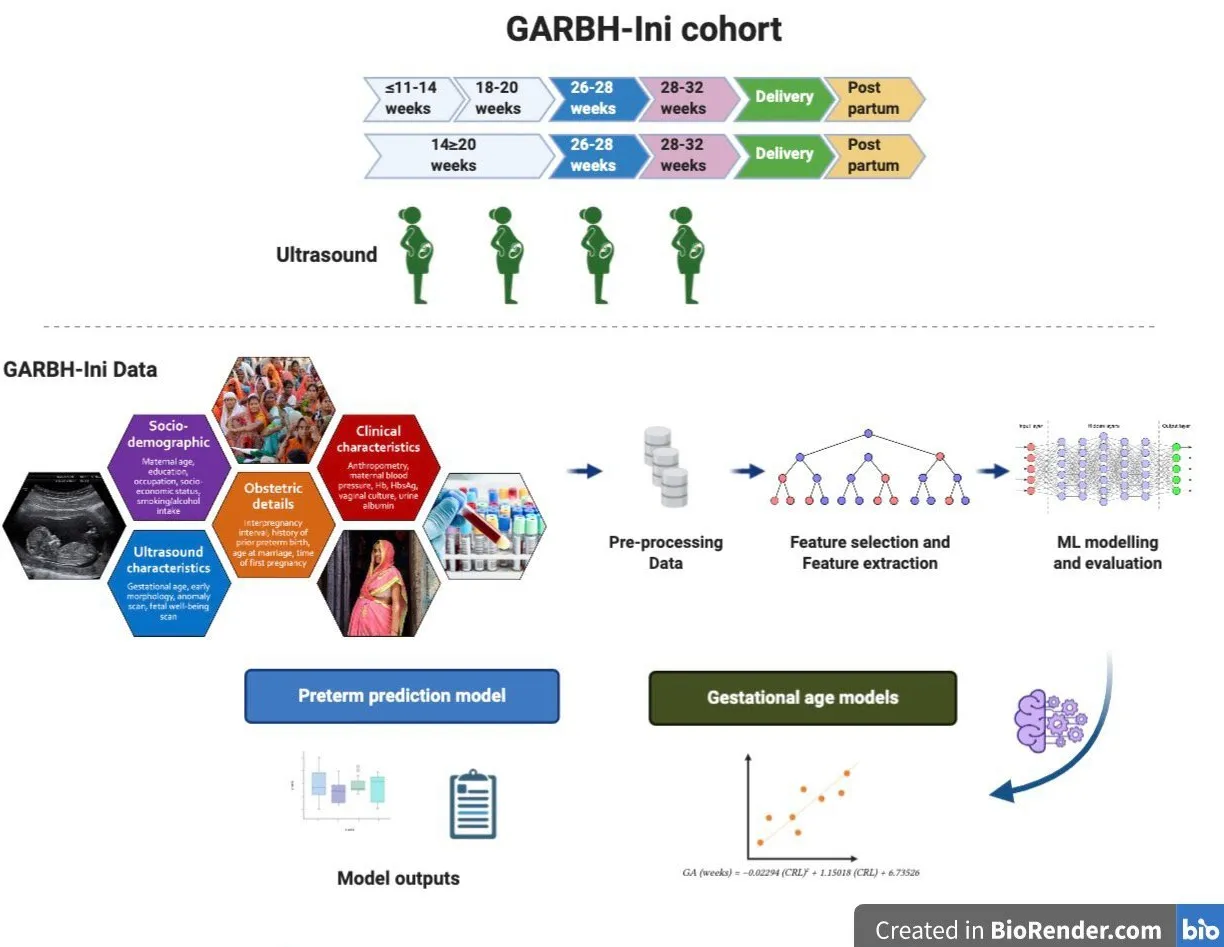
The duration of gestation of pregnancy is the period between the date of conception and date of delivery, which is about 40 weeks of gestation. Estimating gestational age is crucial for accurate prediction of the date of delivery and classifying it as term or preterm, a birth occurring before 37 weeks of gestation. Preterm birth is one of the leading causes of complications for the newborn that can lead to its death. The first trimester, a period between the date of conception and 14 weeks, is known to be the most accurate period to determine the gestational age. Several models have been developed globally to estimate gestational age using the length of fetus measured by ultrasonography in the first trimester of the pregnancy. One of the most common models, the Hadlock model, built on an American population, is predominantly used in India. In this study, we developed an Indian population-specific gestational dating model and compared its performance.
Our study’s dataset comes from GARBH-Ini, an ongoing pregnancy cohort of North Indian women to study preterm births. This study cohort is part of a mission to promote maternal and child health and is one of the five national missions by Government of India under Atal Jai Anusandhan Biotech Mission - Undertaking Nationally Relevant Technology Innovation (UNaTI). GARBH-Ini is funded by the Department of Biotechnology, and is spearheaded by Translational Health Science and Technology Institute (THSTI), Faridabad at Civil Hospital, Gurugram, Haryana. IIT Madras is a data science partner with THSTI, and this data science project is funded under the Grand Challenges India Program by BIRAC, Government of India, and Bill and Melinda Gates Foundation.
GARBH-Ini is a clinical dataset which consists of a large number of features including anthropometric, socioeconomic, clinical, and ultrasonographic parameters. We applied various filtering criteria such as clinical filtering, based on clinically relevant cut-offs or data-driven approaches that use clustering to remove noise. Data-driven filtering method performed better than the standard clinical based filtering. Machine learning algorithms such as generalised linear modelling and random forest were used to identify features that correlate with gestational age in the first trimester. Using this set of selected features, a population-specific model was developed using a polynomial regression-based approach scanning a large list of all possible combinations of these features. Garbhini-GA1 formula, a quadratic polynomial based on the length of the fetus, was selected which performed best in the unseen test dataset.
We wanted to then ask if this Garbhini-GA1 formula performed better than Hadlock formula (used by default) for Indian population. Given that both Hadlock and Garbhini-GA1 formulae are based on the length of the fetus, there was performance parity in terms of gestational age estimation. An accurate estimation of gestational age is crucial for the management of preterm birth. Garbhini-GA1, the first such formula developed in an Indian setting, estimates preterm birth rates with higher accuracy, especially.
A strength of using the Garbhini-GA1 formula is that it caters to the lack of representativeness of an Indian population in published models used worldwide. Further, the data-driven approaches used in several parts of this study have led to a clean dataset to evaluate multiple combinations of several clinical and socio-demographic features. This is an important feature of this study as it reduces imprecision to the minimum that would not have been possible if alternate clinical filtering approaches were used. Such methods have helped in the standardization of fetal length as a primary predictor for the first trimester. The Garbhini-GA1 formula would be useful in serving as a gold standard in estimating gestational age in second and third trimesters where population-based differences have a greater potential in affecting growth. The choice of dating formula influences clinical and epidemiological research that attempts to study preterm birth in lower and middle-income countries. The results in this study will be validated further in other ethnic cohorts in India to increase its potential for broader use in a clinical setting.
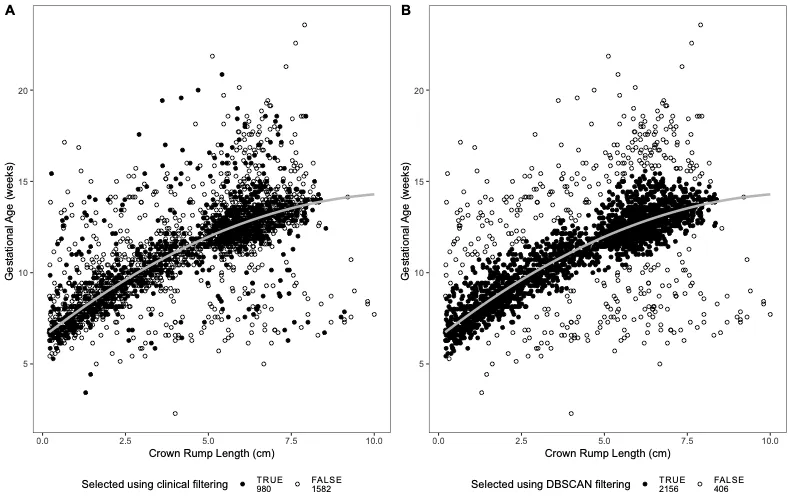
Contributors
Ramya Vijayram, Nikhita Damaraju, Ashley Xavier, Bapu Koundinya Desiraju, Ramachandran Thiruvengadam, Sumit Misra, Shilpa Chopra, Ashok Khurana, Nitya Wadhwa, GARBH-ini, Raghunathan Rengaswamy, Himanshu Sinha, Shinjini Bhatnagar
Article
Vijayram, R., Damaraju, N., Xavier, A., Desiraju, K., Thiruvengadam, R., Misra, S., … & Bhatnagar, S. (2020). Comparison of first trimester dating methods for gestational age estimation and their implication on preterm birth classification in a North Indian cohort. medRxiv, 2019-12.
Keywords
Maternal health, Artificial Intelligence, Machine Learning, Preterm Birth, Health Informatics, Data Science, Healthcare, Perinatal Epidemiology, Biomedical data science
Dataset released
All supplementary data used in the manuscript are submitted. Primary data can be shared according to the GARBH-Ini data sharing policy which is available on request
Media coverage
Acknowledgement
GARBH-Ini is funded by the Department of Biotechnology, and is spearheaded by Translational Health Science and Technology Institute (THSTI), Faridabad at Civil Hospital, Gurugram, Haryana. IIT Madras is a data science partner with THSTI, and this data science project is funded under the Grand Challenges India Program by BIRAC, Government of India, and Bill and Melinda Gates Foundation.