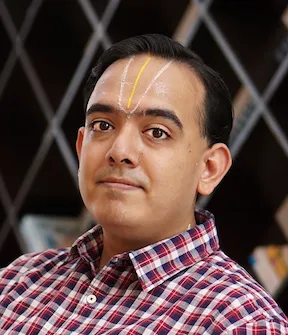
--Dr. Balaji Vasan Srinivasan--
In today’s digital world, social media and websites have become an important tool for communication. As digital communication is becoming more and more important, companies are looking forward to increase their user engagement to advertise their products, agenda or to just spread knowledge. One of the ways to increase user engagement is to provide them with multimodal content which is a combination of two or more modes of communication such as text, image, video, audio etc. Multimodal content have been shown to be more effective and impactful and provides with better understanding. Therefore, AI researchers are scouting for ways by which AI can be employed to generate multimodal content. Dr. Balaji Vasan Srinivasan, Principal Scientist at Adobe Research- India, has been working in this area and was invited to share developments and his learnings as a RBCDSAI Seminar Series talk entitled “Generating tailored multimodal fragments from documents and Videos” on 25th March, 2022.
Dr Srinivasan started the talk by describing his research interests which constituted multimodal content composition, cross media generation and natural language generation. Talking about multimodal content generation problem, he said that the problem is far from solved and therefore there is immense scope in the field. Giving an overview of recent trends, he said that with transformers, understanding of multimodal semantics is picking up and models like CLIP, VILBERT, UNITER are trying to capture the visual and the textual modalities into same space. Next, he talked about his work that entailed three application in multimodal space: Generating a teaser for long document for different readers, generating multimodal answers to questions from a document, generating multimodal fragment for video consumption (making table of content for video.
Talking about the benefits of multimodal teasers, he said they can provide knowledge, promote a brand or alert the audience and one can generate various teasers from same document. The goal of the research was that given unstructured text and image content and a target user need as input, design a system should be able to synthesize multimodal fragments satisfying the need while representing the input content. His research team at Adobe Research solved this problem by assuming that there is a repository of images that can embellish the content that one gets from the input document and so one essentially needs to look at different parts of the input document and try to come up with good collection of images that would go hand in hand with different concepts that are represented in the document. The three key steps involved in this method were need-based retrieval using relevant and diverse queries, need-adapted variants of multimodal fragments (either parallel or non-parallel) and stylistic adaptation of perceptual attribute in image and text. The performance of method was checked by looking at its similarity to textual summary, relevance to the article, relevance to need and diversity and it was found that proposed method could add value to the task of creating multimodal teasers from documents.
Next, he talked about his work which entailed generating multimodal answers to questions on documents. He said that the goal of this study was to extract a multimodal answer from text and images given a collection of input text along with set of related images and a query. He further told that as there were no existing dataset that can cater to this problem and they found that Wikipedia ha text and images in its articles so they used the text of these articles to create textual question answering datasets. They developed two datasets MS Marco and Natural Questions to create Multimodal Input Multimodal Output QA datasets and identified the Question answer pairs coming from Wikipedia paragraphs and scraped the images in those articles which led to collection of some multimodal documents. To assess the method, Dr Srinivasan’s team came up with proxy supervision score which depends on proximity of image to the answer, similarity of the image caption to the answer passage and similarity of image caption to the question. Visual stream was trained using the proxy supervision scores, pre-training was done using conceptual captions dataset and language pre-training. Basically, they extended the UNITER based architecture, pre-trained visual and textual transformer parts with conceptual captions but added a cross attention layer between text and image part. As a baseline they used BERT based question answering model to get question answer and used UNITER based model to find best accompanying image that should go with that. Performance studies showed that cross modal attention helps get better output even with textual modality and the results also showed that proxy scores are good surrogate for image relevance. Dr Srinivasan added that their study was one of the first exploration on modality agnostic question answering.
Next, he discussed his work on developing non-linear media. Describing the intention behind the study, he said that videos are engaging but viewing time is in proportion to the duration of the video and videos are consumed in their original order which is a major problem in longer videos and one can overcome the shortcoming of this linear viewing experience by generating sequence of personalized multimodal fragments. Benefits of such non-linear consumption includes providing an understanding of the content within the video without watching in its entirety, allows for quick navigation to the parts that the viewers are interested by linking to the corresponding segments of the video and personalized consumption of video. Giving an overview of method developed to generate multimodal fragments for non-linear media, Dr Srinivasan listed four steps:
- Extract visual and auditory information from the video
- Create contiguous multimodal clusters
- Select representative multimodal fragment for each cluster
- Re-ordering of fragments to obtain a preference aligned consumption order.
This was tested on different kinds of videos with different duration. Dr Srinivasan concluded by saying that based on baseline evaluations the novel approach for consumption of a video in a non-linear personalized manner showed a good performance on diversity among generated fragments, covering all shots of a given video and serving the personalization needs of the user. The talk was well-received by the audience.
The video is available on our YouTube channel: Link.
Keywords
Multimodal Teasers, Textual Modality