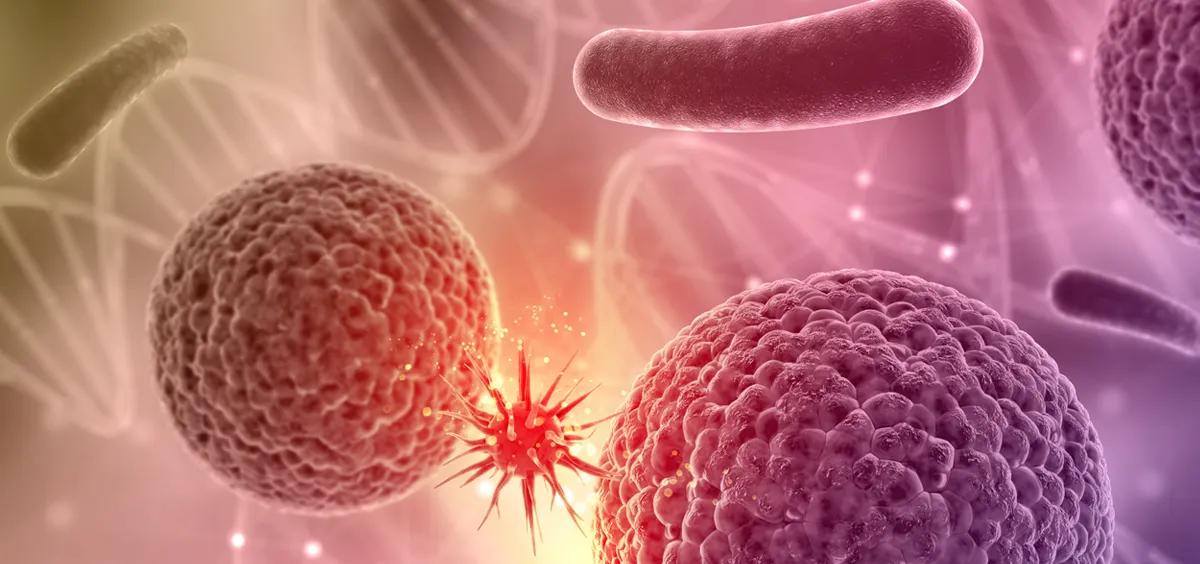
As per the National Cancer Registry Programme Report 2022, over 13 lakhs people in India suffer from cancer each year making India rank third nation-wise in the number of cancer cases across the world. The figures are not good globally as well! As per the WHO, cancer is a leading cause of death worldwide and accounted for nearly one in six deaths in the year 2020. The disease not only torments the patient but is physically, emotionally and financially draining for the family as well.
Cancer is an uncontrolled growth of cells which is caused by alterations/mutations in the cancer-related genes. These alterations in genes can either be due to hereditary or other factors such as smoking tobacco, exposure to radiation, chemical exposure, viruses etc. Currently, the standard cancer treatment includes chemotherapy or radiation therapy that has a range of side effects such as diarrhoea, anaemia, hair loss, appetite loss etc. and in certain cases, a patient succumbs to these side effects. Therefore, the researchers are now trying to identify the cancer-causing genes in an individual so that they can be offered a personalized cancer treatment regime to maximize the efficacy and minimize the side effects.
Dr Karthik Raman, Associate Professor at the Bhupat & Jyoti Mehta School of Biosciences and a core member of the Robert Bosch Centre for Data Science and Artificial Intelligence (RBCDSAI), IIT Madras and her student Malvika Sudhakar decided to work on this problem. They collaborated with Prof. Raghunathan Rengaswamy, Professor at the Department of Chemical Engineering, IIT Madras for the same. The team has come up with an AI-based tool, PIVOT,that predicts the cancer-causing genes akin to an individual. The work has been published in a reputed peer-reviewed journal Frontiers in Genetics.
“Cancer, being a complex disease, cannot be dealt with in a one-treatment-fits-all fashion. As cancer treatment increasingly shifts towards personalised medicine, such tools and models that build toward pinpointing differences between patients can be very useful,” says Dr Raman.
The gene prediction by tool is based on a supervised machine learning model that utilizes information on mutations, expression of genes, copy number variation in genes and perturbations in the biological network due to an altered gene expression to predict the cancer causing genes in a patient.
The tool classifies genes as tumour suppressor genes, oncogenes or neutral genes. The tool was able to successfully predict both the existing oncogenes and tumour-suppressor genes like TP53, PIK3CA etc. and new cancer-related genes such as PRKCA, SOX9 and PSMD4.
Although, there does exist tools that identify personalized cancer genes, however, they use unsupervised learning method and predicts based on the presence and absence of mutations in cancer-related genes. This study, however, is the first one to use the supervised learning method by applying gene-based labelling that is cancer-specific. This new model also takes into account the functional impact of the mutations while making predictions.
“The research area of precision medicine is still at a nascent stage. PIVOT helps push these boundaries and presents prospects for experimental research based on the genes identified,” says Malvika Sudhakar, lead author of the study.
Researchers are currently building AI prediction models for three different types of cancer i.e., breast invasive carcinoma, colon adenocarcinoma, and lung adenocarcinoma but intend to extend it further to many more cancer types. The team is also working on a list of personalized cancer-causing genes that can help in identifying the suitable drug for patients based on their personalized cancer profile.
Contributors
Malvika Sudhakar, Raghunathan Rengaswamy and Karthik Raman
Article
Malvika Sudhakar, Raghunathan Rengaswamy and Karthik Raman, Multi-Omic Data Improve Prediction of Personalized Tumor Suppressors and Oncogenes. In Frontiers in Genetics,13:854190.
Keywords
Machine learning, Driver genes, Personalized driver genes, Cancer genomics, PIVOT