RBCDSAI is committed to the advancement of the society by leveraging AI to solve various social issues. Several socially relevant projects are being carried out at the Centre and some of these are in association with other organizations. This article talks in brief about some of these projects, which strive to make a difference in healthcare, environment, and finance sectors.
A new framework to lend loans in a smarter way
A moneylender, be it a person or a financial institution, always aims to lend their money to a less risky customer who will be able to repay their loan. However, predicting that a customer may default the loan repayment is difficult especially for the microfinance sector whose customers are rural people with no credit history. While many prediction models have been developed in the past to predict the delinquency in loan repayment, financial institutions believe that the financial distress of a household is a more robust indicator and should be predicted or measured while granting a loan. However, predicting financial distress or the over-indebtedness of a household is a much more daunting task than predicting delinquency as it depends on a myriad of factors.
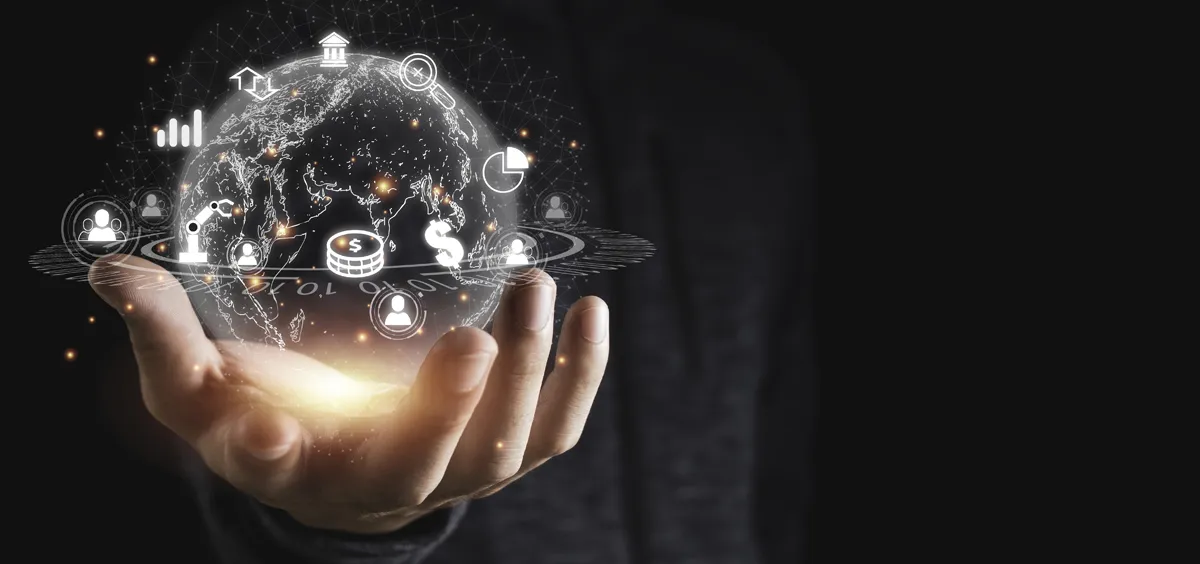
Dr Nandan Sudarsanam and his team at IIT Madras has now been able to develop a framework that can be used to detect the over-indebtedness of a household. To carry out this study, the team analysed the financial data of 200,000 households collected by the Indian microfinance sector company -Dvara KGFS. The team used a sequential modelling framework based on the Positive and Unlabeled (PU) learning approach and employed Recurrent Neural Networks (RNN) to predict the financial stress of a household which may lead to delinquency. The framework developed by the team can be used by the microfinance sector to understand the financial well being of their customers to lend loans more smartly.
Cybercrimes and crime against women increased while other crimes declined during the COVID-19 lockdown
COVID-19 pandemic brought the economic activities to a near standstill as the nations imposed lockdown to curb the spread of infection. Economically desperate times are precursors for the rise in criminal activities, however, the restricted movement of people and criminals allowed during the raging phases of the pandemic begged a study to find out if the cases of crime increased or declined during the phases of complete or partial lockdowns.
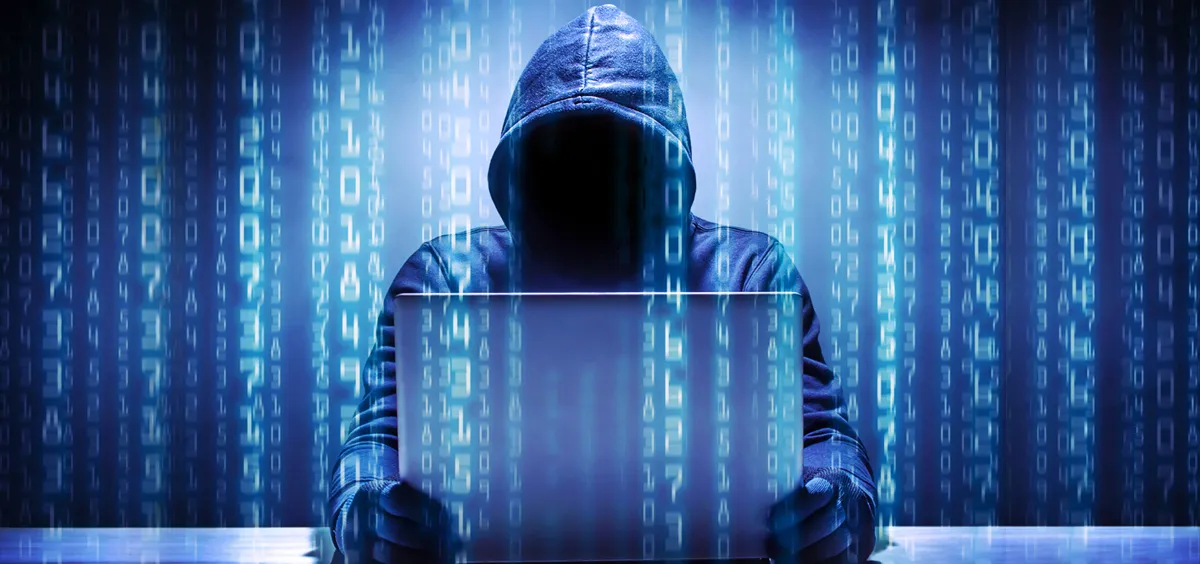
Now, a recent study by Nandan Sudarsanam and his team at IIT Madras and Robert Bosch Centre of Data Science and Artificial Intelligence (RBCDSAI) have found that though vehicle and traffic violations, property offences and missing person cases were considerably low when the movement of people was restricted, however, the incidences of cybercrimes, bank/ATM frauds and crime against women and elderly increased during the complete and partial lockdown periods.
To carry out this study, the team used the crime data received from the State Crime Records Bureau and State Police Master Control Room of Tamil Nadu. They studied the crime rates before complete lockdown, during the complete lockdown, during the partial lockdown and a period when all the restrictions were lifted using the Bayesian time series model for different categories of crimes. The mismatch between the distress calls and actual reporting of crime shows that restricted movement of people limited their ability to formally report a crime. The study calls for urgent actions to ensure that people have easy access to police for reporting crimes during such desperate times when stay-at-home orders are imposed by the government.
How COVID-19 impacts population movement: A data-driven analysis to study population behavior during a pandemic
Ever since the COVID-19 outbreak, enough emphasis has been laid on social distancing. Scientific evidence available on the transmission of SARS-CoV-2 shows that, the disease spreads through droplets launched from an infected person, via coughing, sneezing, or talking, that land on a healthy person in close proximity (less than 6 feet). Epidemiological researchers have found social distancing measures to be highly effective in containing the spread of a virus in the absence of a proven cure or vaccine. So, researchers at RBCDSAI, IITM attempted to measure statistical significance of such non pharmaceutical interventions, and how adherence to stay at home orders affects the COVID-19 epidemic growth rate.
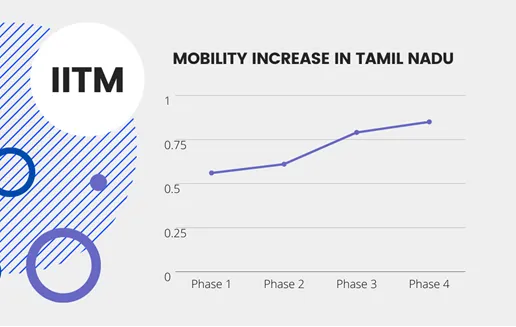
In partnership with Facebook Data for Good, Facebook’s user location data was analysed to study the population behaviour of India, and particularly Tamil Nadu, during COVID-19 lockdown. We built pipelines to process the enormous amounts of data and created situation reports daily. Our team used various data analysis tools to process the datasets, and automated data wrangling and creation of situation reports. Using optimized pipelines and process automation, we were able to stay up-to-date on the COVID-19 situation in Tamil Nadu. The situation reports were used by the government to frame relevant policies, predict what could be the next hotspot and allocate resources. Although this work was targeted at Tamil Nadu data specifically it can be generalized to any area in India.
Read more about the analysis here.
Data Science and IoT for addressing ambient air sanctity
Titled ”Kaatru,”(Tamil word for Air) the project is aimed at mapping ambient environmental conditions, which are highly resolved both spatially and temporally. Currently, monitoring stations are installed at fixed locations and are sparsely distributed, owing to excessive costs associated with deployment and maintenance.
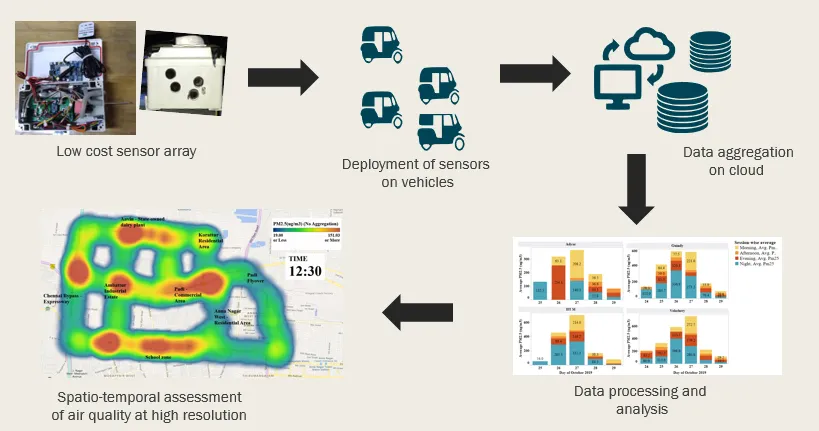
The proposed project involves building a network of low cost, vehicle mounted environmental monitoring devices which would crisscross the city. Modular hardware design allows for easy swapping and upgradation of the sensors. Each device has 7 sensors that can measure up to 25 environmental parameters ranging from air quality information to solar intensity and even road condition. These distributed units would be capable of collecting environmental data with unprecedented spatial resolution. These IoT units are location aware and have bidirectional communication with a central server, sending data samples once every 20 seconds. This near real-time, high-resolution data, combined with analytical tools, would offer deeper insights on location specific environmental conditions prevailing within a city. The objective of project Kaatru is to leverage Big Data Analytics, Data Science, Artificial Intelligence and IoT to provide hyperlocal environmental insights and develop solutions for social and commercial impact. The goal is to obtain high resolution, pan-India environmental data, that can be an incredible resource for researchers working in data science to answer many questions of practical social significance.
Read more about the project here.
Machine learning helps find genes responsible for cancer
Cancer, on diagnosis, can completely change the life of the person involved. There is a lot of emotional and physical trauma involved in the process of treatment, which at times doesn’t help. Combating cancer needs a better understanding of the driving force behind it. A cancer cell mutates to form a tumour, the genetic make-up of which varies across individuals, tissues and time. Identification of driver genes and driver mutations is essential to understand tumorigenesis. At the Computational Systems Biology Lab (CSBL) at IIT Madras, we were interested in the identification of the driver genes.
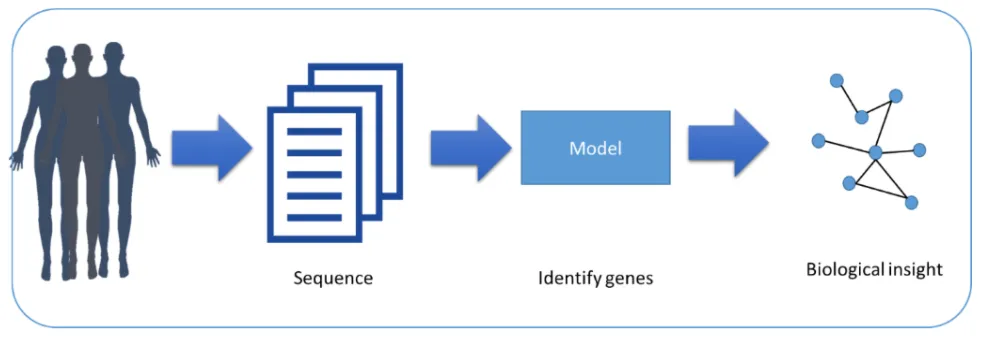
A cell accumulates mutations in the driver genes. With the advent of technology, the volume of cancer data available publicly, for various cancer types, has gone up. Analyzing this data helps in extracting information on driver genes. In our study, we classify driver genes as Tumour Suppressor Genes (TSGs) and oncogenes (OGs) based on their functionalities and build models. These pan cancer models are then used to identify novel driver genes. When presented with breast cancer data the model successfully identifies tissue specific genes. A lot more aspects are being taken into consideration to build a more robust model.
Using AI to improve maternal health outcomes by increasing program engagement
India accounts for 12% of maternal deaths, and 16% of child deaths happening globally. With a population of over a billion, India had more than 820,000 child deaths in 2019. Majority of these deaths were preventable and can be accounted to information asymmetry. Thus, there is an immediate need to leverage Information and Communication Technologies to mitigate pregnancy related risks, and to improve maternal health by closing the information gaps. In collaboration with Google Research and ARMMAN, a non-profit based in India, this project aims to further the use of call-based information programs. The program uses cell phone calls to regularly disseminate health related information, and has proven to affect health parameters positively. Therefore, there is a need for early identification of women who might not engage in these programs
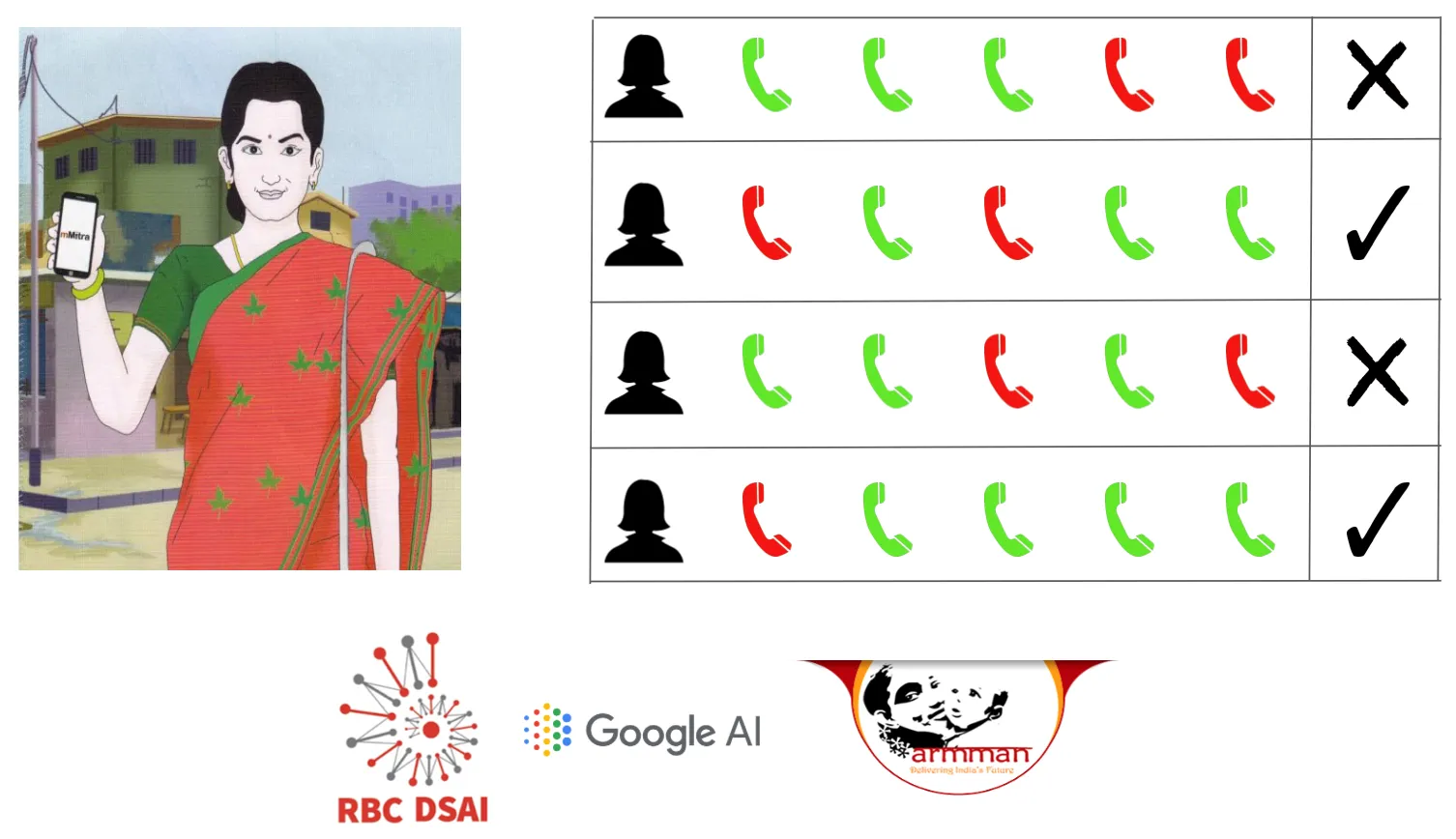
Anonymized call records of over 300,000 women, registered in an awareness program created by ARMMAN, were analyzed. Using robust deep learning based models, short term and long term dropout risks were predicted based on call logs and beneficiaries’ demographic information. When compared with other methods our model improved the accuracy of short-term and long term forecasting by 13% and 7% respectively. The applicability of this method in the real world through a pilot validation that uses our method to perform targeted interventions is being discussed.
IndicBERT: A multilingual language model for 11 regional Indian languages
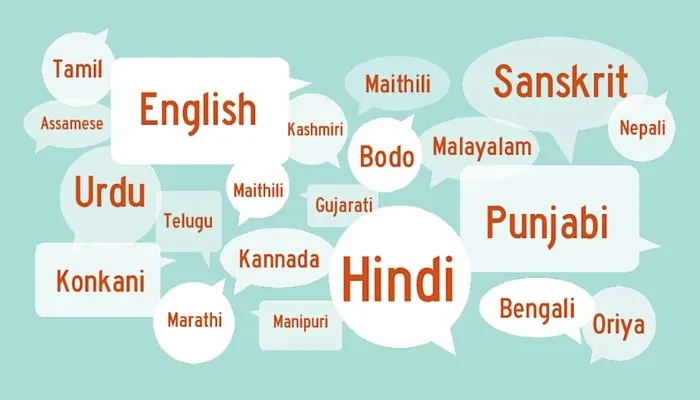
Indian languages are widely spoken around the world by more than a billion speakers, and yet the NLP ecosystem for Indian languages is poorly developed - lacking in terms of both datasets and models for various NLP tasks. Researchers at RBCDSAI worked on creating quality large-scale datasets and developing several foundational NLP models for 11 major Indian languages. As part of this work, a general-purpose natural language understanding model, called IndicBERT was released. The model can perform a wide variety of NLP tasks like sentiment analysis, common-sense reasoning, question answering etc. on Indic languages. The resources that were built can accelerate both research and development around Indic languages and can further the reach of NLP technology to the masses.
Estimation of gestational age in the Garbh-INI cohort
An accurate time of conception is not known in a majority of naturally occurring pregnancies. The time of conception is vital to estimate the age of the foetus (gestational age), the due date and scheduling the various doctor visits to monitor the health of the foetus. The predicted due date also impacts the classification of birth as preterm or term. Different models have been developed globally to estimate gestational age by ultrasonography in the first trimester of pregnancy. The Hadlock model is what is predominantly used in India but it was built entirely on a western population. Some newer models include Indian populations for model building but the contributions are low. In this study, we develop an Indian population-specific dating formula and compare its performance with published formulae.
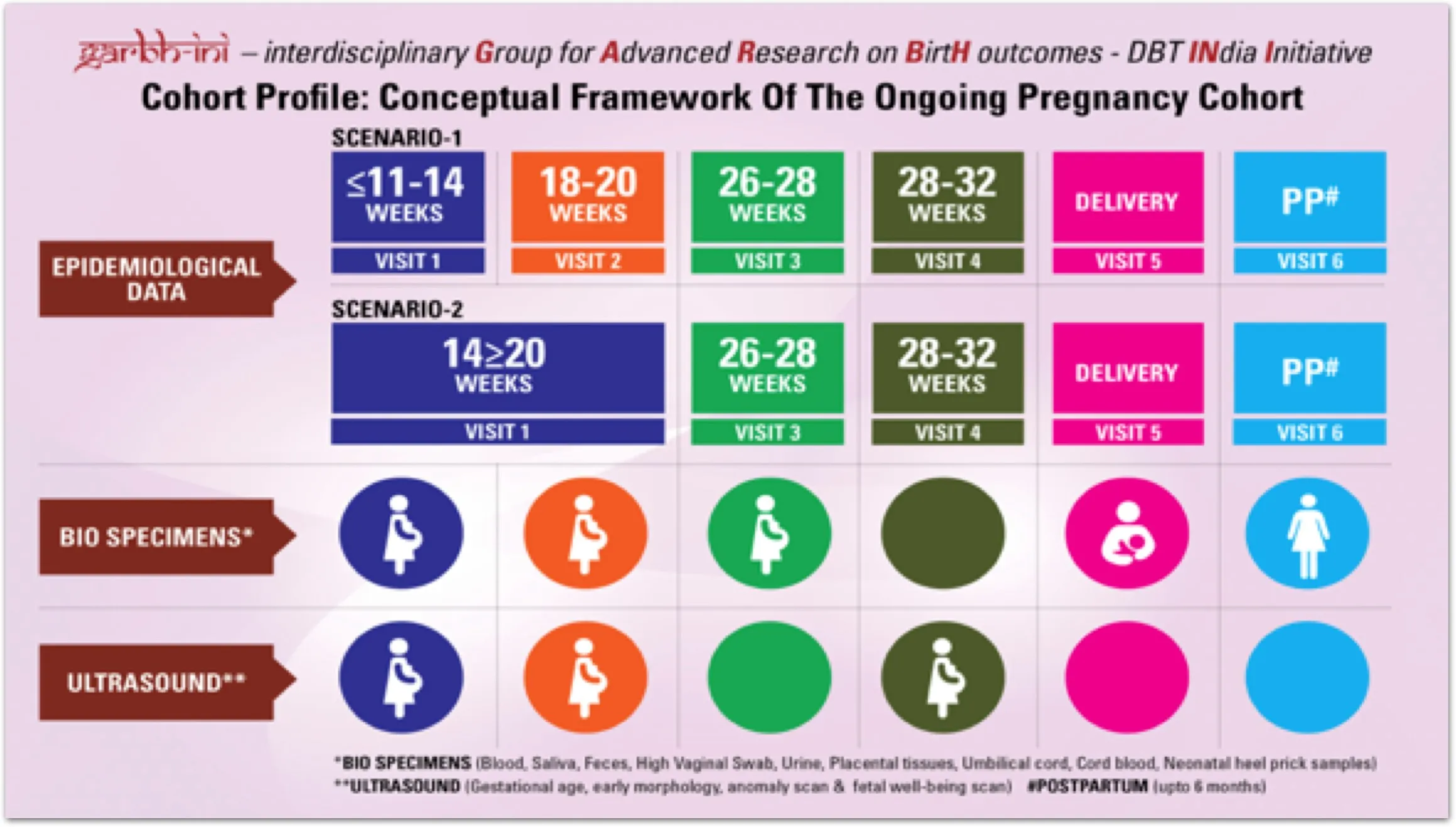
We observed that the conventional clinical criteria used to remove outliers in the data had poor discriminatory power in our cohort, we then proceeded to utilize data-driven approaches such as density-based clustering to remove outliers. We applied advanced machine learning algorithms to identify relevant features for GA estimation and developed an Indian population-specific formula (Garbhini-GA1) for the first trimester. We found that Crown-Rump Length (CRL) was the most crucial parameter in estimating GA and no other clinical or socioeconomic features contributed to GA estimation.
Performance of Garbhini-GA1 formula, a non-linear function of CRL, was at least equivalent to existing models for estimation of the first trimester, if not, more appropriate for use in an Indian population. An accurate estimation of GA is crucial for the management of preterm birth. Garbhini-GA1, the first such formula developed in an Indian setting, estimates PTB rates with higher accuracy, especially when compared to commonly used Hadlock formula. Our results, therefore, reinforce the need to develop population-specific gestational age formulae.