Scaling graph representation learning algorithms in an implementation agnostic fashion
Apr 18, 2022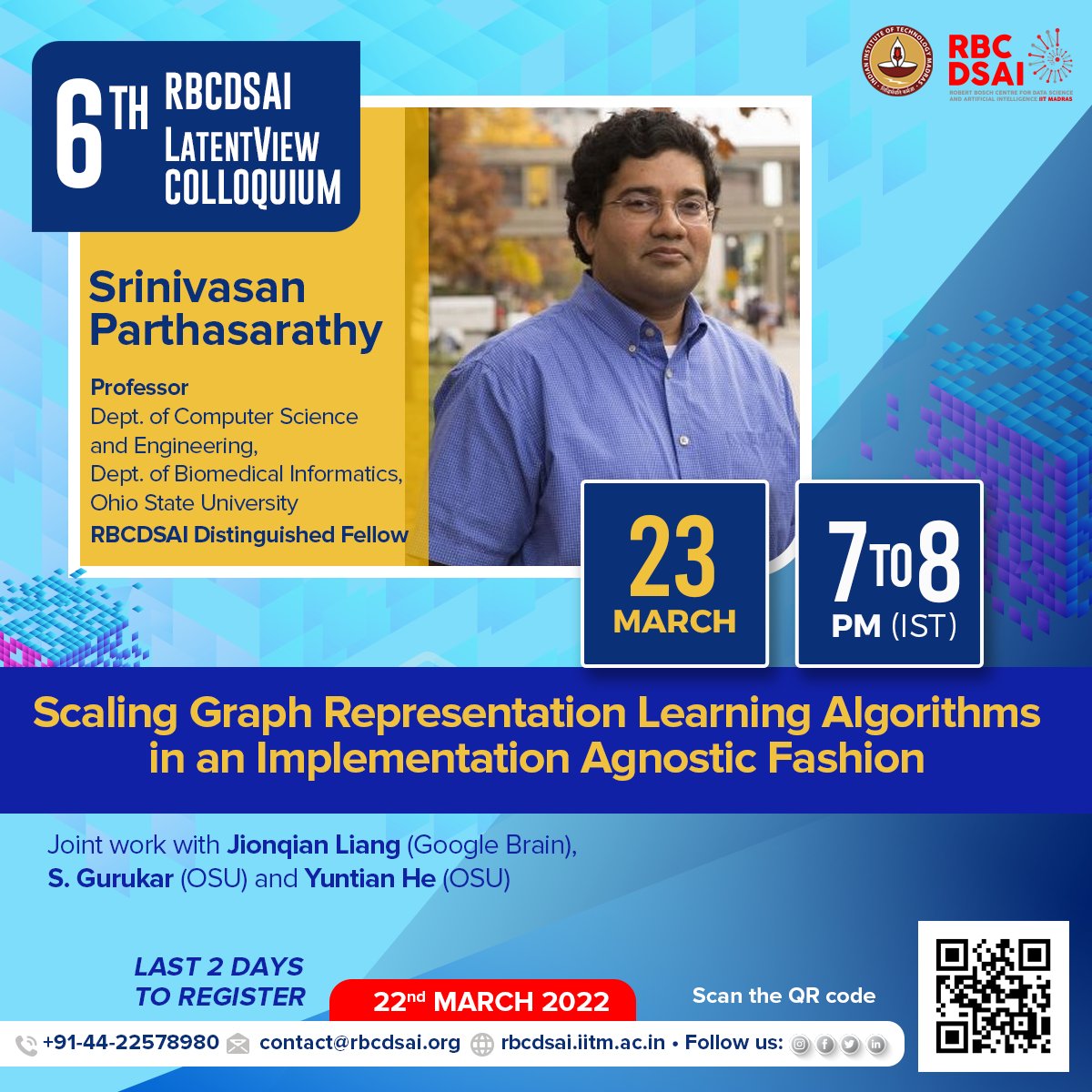
Scaling graph representation learning algorithms in an implementation agnostic fashion
--Prof. Srinivasan Parthasarathy--
A graph, which consists of nodes and edges, is a pictorial representation of the relationship between various objects. Analysis of graphs can help in inferring social, biological, road, finance and other networks as well. Prof. Srinivasan Parthasarathy who is Professor at Dept. of Computer Science and Engineering and Department of Biomedical Informatics at the Ohio State University and RBCDSAI Distinguished Fellow gave a talk on his research in the area of graph analysis through a talk titled “Scaling graph representation learning algorithms in an implementation agnostic fashion” which was organized as a part of Sixth RBCDSAI Latent View Colloquium on 23rd March 2022.
3 min read
Accurate and Interpretable AI models: Towards Deployable AI
A self-driving car bumps into a lamppost. A doctor prescribes the wrong treatment to a patient based on an AI-based diagnostic tool. A missile is misfired by an AI-based defense system. An unfair decision provided by a banking chatbot leads to loss of customers.
The above examples are probably sufficient to explain why there is a need to tread carefully while deploying AI-based solutions in the real world. While wrong predictions made by recommendation systems in domains like retail might be inexpensive, such predictions in domains like healthcare, self-driving vehicles, banking or defense can cause hefty monetary losses or even loss of lives.
3 min read
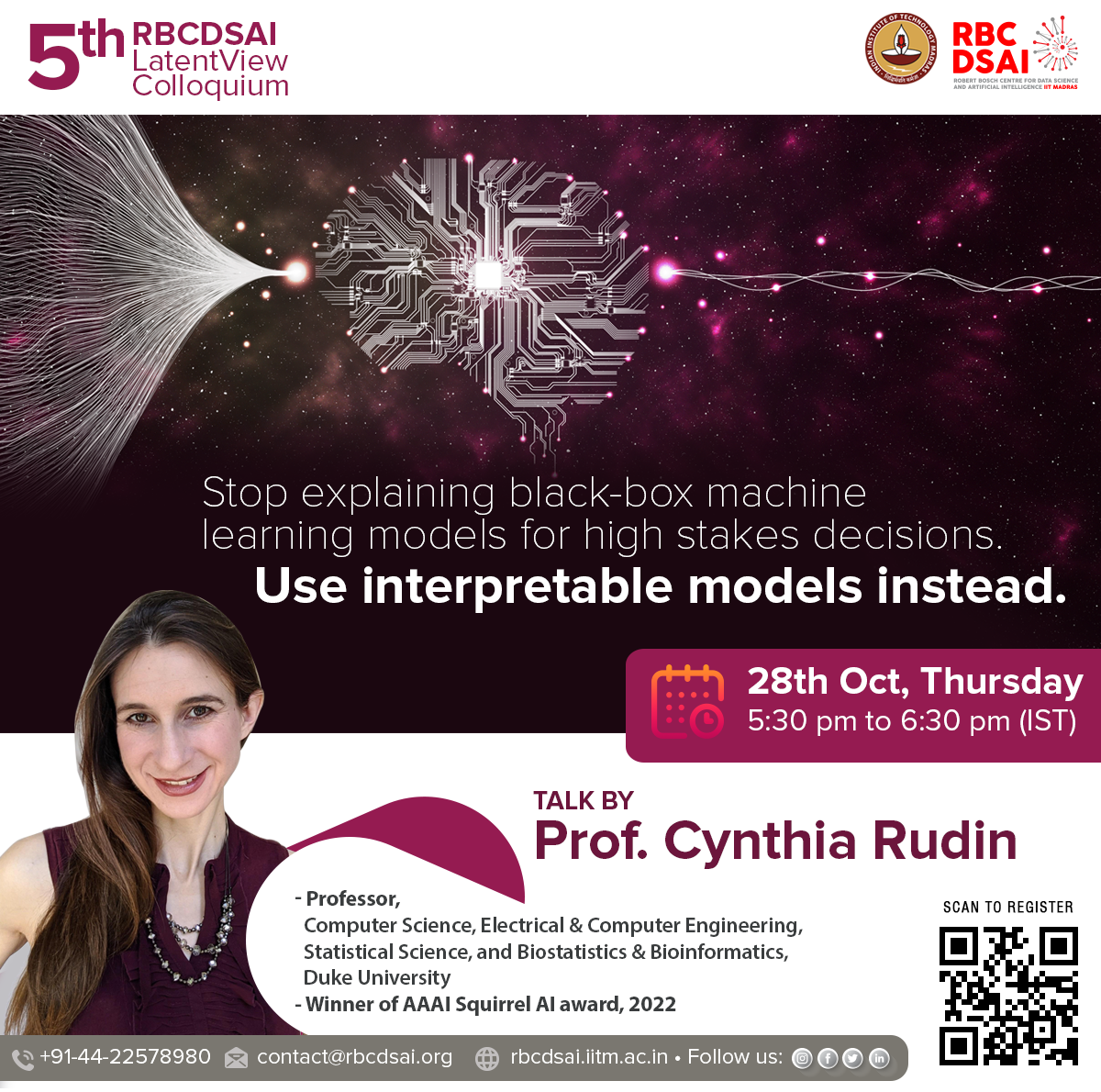
Interpretable AI
--Prof. Cynthia Rudin--
For quite a long time, researchers and the public were thrilled by the wonders of machine learning. However, over the period of time, the community realized that the machine learning models aren’t a magic wand and they are as best as the data provided to them during the training and development stage. As the world started making decisions based on AI, there were soon conflicts between human and machine intelligence and therefore the need for explainability of black-box models become apparent.
5 min read
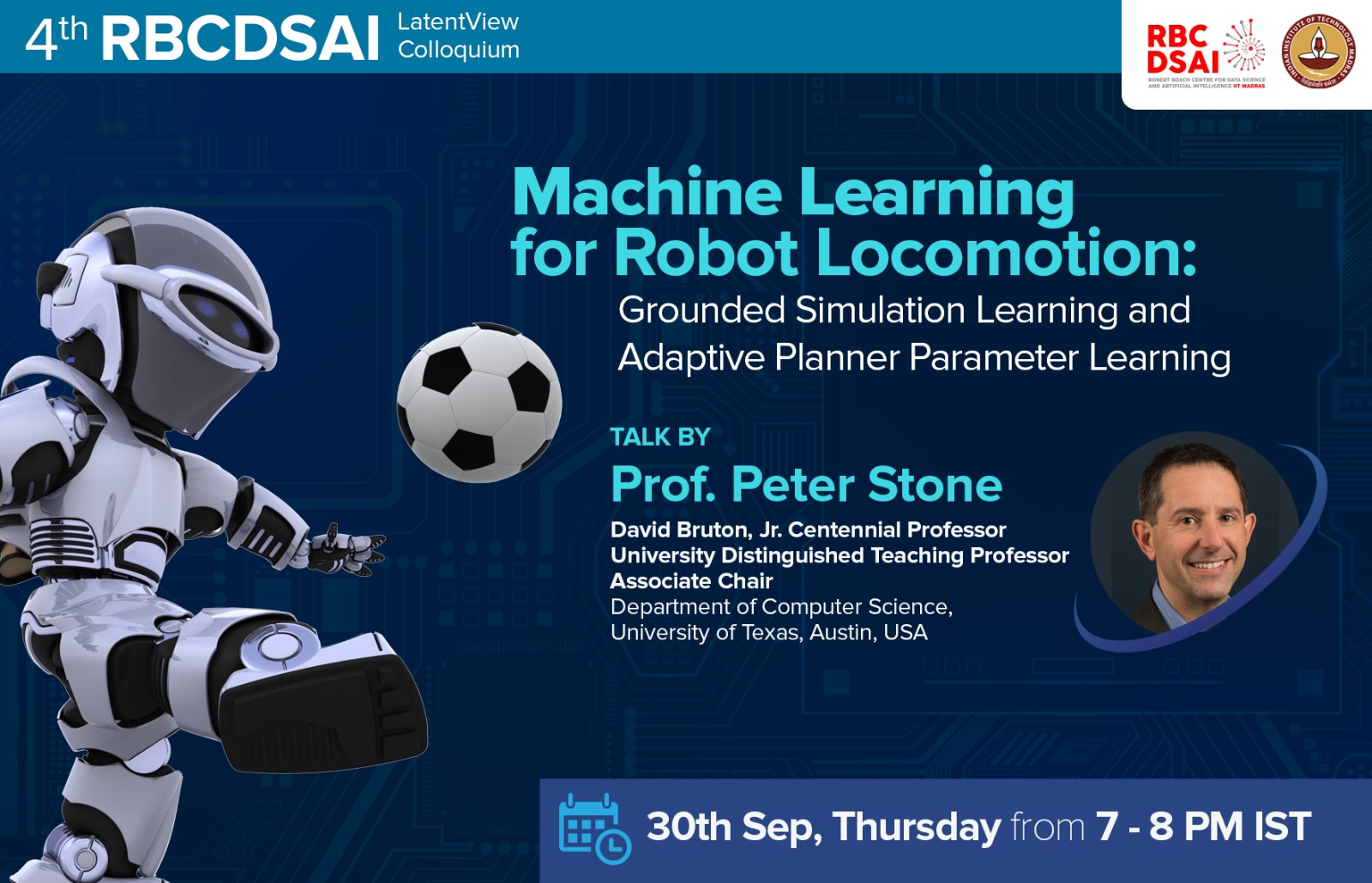
Machine Learning for Robot Locomotion: Grounded Learning and Adaptive Parameter Learning
--Dr.Peter Stone--
Robot locomotion continues to remain a challenging problem in spite of the advances in the field. To gain more insights on the topic, a talk on “Machine Learning for Robot Locomotion: Grounded Learning and Adaptive Parameter Learning” was organized as a part of the fourth latent colloquium on 30th September 2021. The talk was delivered by Prof. Peter Stone, who is the David Bruton, Jr. Centennial Professor and Associate Chair of Computer Science, as well as Director of Texas Robotics, at the University of Texas at Austin.
3 min read